180 câu trắc nghiệm Kinh tế lượng – Phần 6
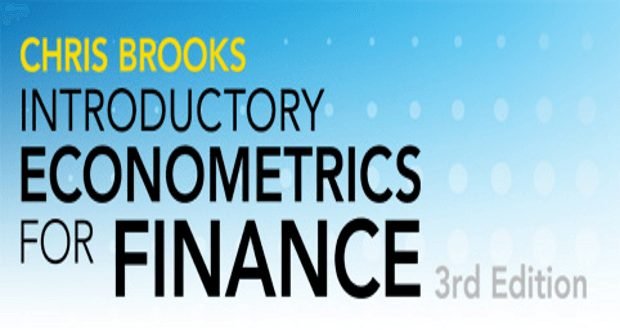
Chapter 16: Additional Topics in Time Series Regression
KTL_001_C16_1: If Yt is I(2), then
● \({\Delta ^2}{Y_t}\) is stationary.
○ Yt has a unit autoregressive root.
○ \(\Delta {Y_t}\) is stationary.
○ Yt is stationary.
KTL_001_C16_2: A VAR with five variables, 4 lags and constant terms for each equation will have a total of
○ 21 coefficients.
○ 100 coefficients.
● 105 coefficients.
○ 84 coefficients.
KTL_001_C16_3: The order of integration
○ can never be zero.
● is the number of times that the series needs to be differenced for it to be stationary.
○ is the value of \({\phi _1}\) in the quasi difference \(\Delta {Y_t} – {\phi _1}{Y_{t – 1}}\).
○ depends on the number of lags in the VAR specification.
KTL_001_C16_4: The following is not an appropriate way to tell whether two variables are cointegrated
● see if the two variables are integrated of the same order.
○ graph the series and see whether they appear to have a common stochastic trend.
○ perform statistical tests for cointegration.
○ use expert knowledge and economic theory.
KTL_001_C16_5: ARCH and GARCH models are estimated using the
○ OLS estimation method.
● the method of maximum likelihood.
○ DOLS estimation method.
○ VAR specification.
KTL_001_C16_6: A VAR with k time series variables consists of
● k equations, one for each of the variables, where the regressors in all equations are lagged values of all the variables
○ a single equation, where the regressors are lagged values of all the variables
○ k equations, one for each of the variables, where the regressors in all equations are never more than one lag of all the variables
○ k equations, one for each of the variables, where the regressors in all equations are current values of all the variables
KTL_001_C16_7: The BIC for the VAR is
○ \(BIC\left( p \right) = \ln \left[ {\det \left( {{{\hat \Sigma }_u}} \right)} \right] + k\left( {kp + 1} \right)\frac{2}{T}\)
○ \(BIC\left( p \right) = \ln \left[ {\det \left( {{{\hat \Sigma }_u}} \right)} \right] + k\left( {p + 1} \right)\frac{{\ln \left( T \right)}}{T}\)
● \(BIC\left( p \right) = \ln \left[ {\det \left( {{{\hat \Sigma }_u}} \right)} \right] + k\left( {kp + 1} \right)\frac{{\ln \left( T \right)}}{T}\)
○ \(BIC\left( p \right) = \ln \left[ {SSR\left( p \right)} \right] + k\left( {kp + 1} \right)\frac{{\ln \left( T \right)}}{T}\)
KTL_001_C16_8: The lag length in a VAR using the BIC proceeds as follows: Among a set of candidate values of p, the estimated lag length \({\hat p}\) is the value of p
○ For which the BIC exceeds the AIC
○ That maximizes BIC(p)
○ Cannot be determined here since a VAR is a system of equations, not a single one
● That minimizes BIC(p)
KTL_001_C16_9: The dynamic OLS (DOLS) estimator of the cointegrating coefficient, if Yt and Xt are cointegrated,
○ is efficient in large samples
○ statistical inference about the cointegrating coefficient is valid
○ the t-statistic constructed using the DOLS estimator with HAC standard errors has a standard normal distribution in large samples
● all of the above
KTL_001_C16_10: The EG-ADF test
○ Is the similar to the DF-GLS test
● Is a test for cointegration
○ Has as a limitation that it can only test if two variables, but not more than two, are cointegrated
○ Uses the ADF in the second step of its procedure