180 câu trắc nghiệm Kinh tế lượng – Phần 3
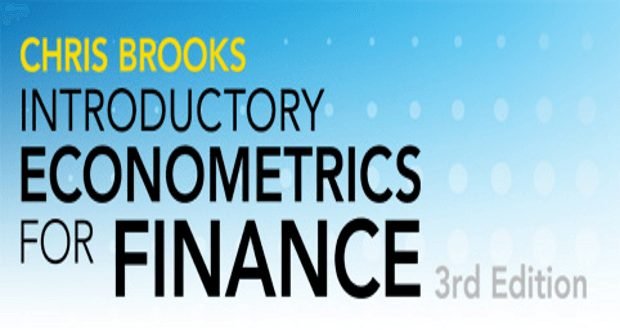
Chapter 7: Hypothesis Tests and Confidence Intervals in Multiple Regression
KTL_001_C7_1: When testing joint hypothesis, you should
○ use t-statistics for each hypothesis and reject the null hypothesis is all of the restrictions fail
○ use the F-statistic and reject all the hypothesis if the statistic exceeds the critical value
○ use t-statistics for each hypothesis and reject the null hypothesis once the statistic exceeds the critical value for a single hypothesis
● use the F-statistics and reject at least one of the hypothesis if the statistic exceeds the critical value
KTL_001_C7_2: In the multiple regression model, the t-statistic for testing that the slope is significantly different from zero is calculated
● by dividing the estimate by its standard error.
○ from the square root of the F-statistic.
○ by multiplying the p-value by 1.96.
○ using the adjusted \({R^2}\) and the confidence interval.
KTL_001_C7_3: If you wanted to test, using a 5% significance level, whether or not a specific slope coefficient is equal to one, then you should
● subtract 1 from the estimated coefficient, divide the difference by the standard error, and check if the resulting ratio is larger than 1.96.
○ add and subtract 1.96 from the slope and check if that interval includes 1.
○ see if the slope coefficient is between 0.95 and 1.05.
○ check if the adjusted \({R^2}\) is close to 1.
KTL_001_C7_4: When there are two coefficients, the resulting confidence sets are
○ rectangles
● ellipses
○ squares
○ trapezoids
KTL_001_C7_5: All of the following are true, with the exception of one condition:
○ a high \({R^2}\) or \({\bar R^2}\) does not mean that the regressors are a true cause of the dependent variable
○ a high \({R^2}\) or \({\bar R^2}\) does not mean that there is no omitted variable bias
● a high \({R^2}\) or \({\bar R^2}\) always means that an added variable is statistically significant
○ a high \({R^2}\) or \({\bar R^2}\) does not necessarily mean that you have the most appropriate set of regressors
KTL_001_C7_6: You have estimated the relationship between test scores and the student-teacher ratio under the assumption of homoskedasticity of the error terms. The regression output is as follows: \({\hat Y}\) = 698.9 – 2.28*STR , and the standard error on the slope is 0.48. The homoskedasticity-only “overall” regression F- statistic for the hypothesis that the Regression \({R^2}\) is zero is approximately
○ 0.96
○ 1.96
● 22.56
○ 4.75
KTL_001_C7_7: Consider a regression with two variables, in which \({X_{1i}}\) is the variable of interest and \({X_{2i}}\) is the control variable. Conditional mean independence requires
● \(E\left( {{u_i}|{X_{1i}},{X_{2i}}} \right) = E\left( {{u_i}|{X_{2i}}} \right)\)
○ \(E\left( {{u_i}|{X_{1i}},{X_{2i}}} \right) = E\left( {{u_i}|{X_{1i}}} \right)\)
○ \(E\left( {{u_i}|{X_{1i}}} \right) = E\left( {{u_i}|{X_{2i}}} \right)\)
○ \(E\left( {{u_i}} \right) = E\left( {{u_i}|{X_{2i}}} \right)\)
KTL_001_C7_8: The homoskedasticity-only F-statistic and the heteroskedasticity-robust F-statistic typically are
○ the same
● different
○ related by a linear function
○ a multiple of each other (the heteroskedasticity-robust F-statistic is 1.96 times the homoskedasticity-only F-statistic)
KTL_001_C7_9: Consider the following regression output where the dependent variable is testscores and the two explanatory variables are the student-teacher ratio and the percent of English learners: \({\hat Y}\) = 698.9 – 1.10*STR – 0.650*EL . You are told that the t-statistic on the student-teacher ratio coefficient is 2.56. The standard error therefore is approximately
○ 0.25
○ 1.96
○ 0.650
● 0.43
KTL_001_C7_10: The critical value of \({F_{4,\infty }}\) at the 5% significance level is
○ 3.84
● 2.37
○ 1.94
○ Cannot be calculated because in practice you will not have infinite number of observations