112 câu trắc nghiệm Kinh tế lượng – Phần 2
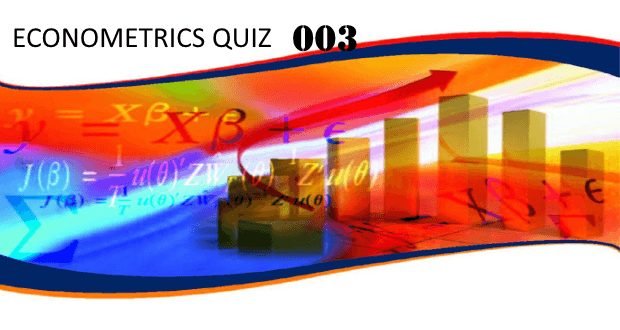
7. Data preparation and preliminary data analysis
KTL_003_C7_1: Most market research studies can be solved only by collecting secondary data.
○ True
● False
KTL_003_C7_2: Which of the following steps is not involved in fieldwork?
○ Selection of fieldworkers
○ Training of fieldworkers
○ Supervision of fieldworkers
○ Evaluation of fieldworkers
○ All of the above
● None of the above
KTL_003_C7_3: Probing helps in motivating the respondent and helps focus on a specific issue.
● True
○ False
KTL_003_C7_4: Which of the following is not an appropriate probing technique?
○ Repeating the question
○ Repeating the respondents’ reply
● Forcing the respondent to remember
○ Eliciting clarification
○ Using objective/neutral questions or comments
KTL_003_C7_5: One of the major editing problem concerns with faking of an interview.
● True
○ False
KTL_003_C7_6: How can a researcher avoid and cross-check for fake interviews?
○ Use complex scales
○ Use dichotomous questions
○ Use only close ended questions
● Use few open-ended questions
KTL_003_C7_7: What types of questions are relatively hard to code?
○ Multiple choice questions
○ Dichotomous questions
● Open-ended questions
○ Likert scale based questions
KTL_003_C7_8: Data cleaning involves which of the following.
○ Substituting missing value with a neutral value
○ Substituting an imputed response by following a pattern of respondent’s other responses
○ Casewise deletion
○ Pairwise deletion
● All of the above
○ None of the above
KTL_003_C7_9: Categorical variables involve what of the following scales?
● Nominal and ordinal
○ Nominal and interval
○ Nominal and ratio
○ Ordinal and ratio
○ Ordinal and interval
○ Interval and ratio
KTL_003_C7_10: Categorical variables involve what of the following scales?
○ Nominal and ordinal
○ Nominal and interval
○ Nominal and ratio
○ Ordinal and ratio
● Ordinal and interval
○ Interval and ratio
KTL_003_C7_11: Discuss data cleaning and its importance in preliminary data analysis.
Data cleaning focuses on error detection and consistency checks as well as treatment of missing responses. The first step in the data cleaning process is to check each variable for data that are out of the range or as otherwise called logically inconsistent data. Such data must be corrected as they can hamper the overall analysis process.
Most advance statistical packages provide an output relating to such inconsistent data. Inconsistent data must be closely examined as sometimes they might not be inconsistent and be representing legitimate response.
In most surveys, it happens so that respondent has either provided ambiguous response or the response has been improperly recorded. In such cases, missing value analysis is conducted for cleaning the data. If the proportion of missing values is more than 10%, it poses greater problems. There are four options for treating missing values: (a) substituting missing value with a neutral value (generally mean value for the variable); (b) substituting an imputed response by following a pattern of respondent’s other responses; (c) casewise deletion, in which respondents with any missing responses are discarded from the analysis and (d) pairwise deletion, wherein only the respondents with complete responses for that specific variable are included. The different procedures for data cleaning may yield different results and therefore, researcher should take utmost care when cleaning the data. The data cleaning should be kept at a minimum if possible.
KTL_003_C7_12: Explain data editing and coding process in details.
The usual first step in data preparation is to edit the raw data collected through the questionnaire. Editing detects errors and omissions, corrects them where possible, and certifies that minimum data quality standards have been achieved. The purpose of editing is to generate data which is: accurate; consistent with intent of the question and other information in the survey; uniformly entered; complete; and arranged to simplify coding and tabulation.
Sometimes it becomes obvious that an entry in the questionnaire is incorrect or entered in the wrong place. Such errors could have occurred in interpretation or recording. When responses are inappropriate or missing, the researcher has three choices:
(a) Researcher can sometimes detect the proper answer by reviewing the other information in the schedule. This practice, however, should be limited to those few cases where it is obvious what the correct answer is.
(b) Researcher can contact the respondent for correct information, if the identification information has been collected as well as if time and budget allow.
(c) Researcher strike out the answer if it is clearly inappropriate. Here an editing entry of ‘no answer’ or ‘unknown’ is called for. This procedure, however, is not very useful if your sample size is small, as striking out an answer generates a missing value and often means that the observation cannot be used in the analyses that contain this variable.
One of the major editing problem concerns with faking of an interview. Such fake interviews are hard to spot till they come to editing stage and if the interview contains only tick boxes it becomes highly difficult to spot such fraudulent data. One of the best ways to tackle the fraudulent interviews is to add a few open-ended questions within the questionnaire. These are the most difficult to fake. Distinctive response patterns in other questions will often emerge if faking is occurring. To uncover this, the editor must analyse the instruments used by each interviewer.
Coding involves assigning numbers or other symbols to answers so the responses can be grouped into a limited number of classes or categories. Specifically, coding entails the assignment of numerical values to each individual response for each question within the survey. The classifying of data into limited categories sacrifices some data detail but is necessary for efficient analysis. Instead of requesting the word male or female in response to a question that asks for the identification of one’s gender, we could use the codes ‘M’ or ‘F’. Normally this variable would be coded 1 for male and 2 for female or 0 and 1. Similarly, a Likert scale can be coded as: 1 = strongly disagree; 2 = disagree; 3 = neither agree nor disagree; 4 = agree and 5 = strongly agree. Coding the data in this format helps the overall analysis process as most statistical software understand the numbers easily. Coding helps the researcher to reduce several thousand replies to a few categories containing the critical information needed for analysis. In coding, categories are the partitioning of a set; and categorization is the process of using rules to partition a body of data.
KTL_003_C7_13: Why should a researcher do a normality and outliers assessment before hypotheses testing?
To conduct many advance statistical techniques, researchers have to assume that the data provided is normal (means it is symmetrical on a bell curve) and free of outliers. In simple terms, if the data was plotted on a bell curve, the highest number of data points will be available in the middle and the data points will reduce on either side in a proportional fashion as we move away from the middle. Normality and outliers analysis provides clarity with regard to fundamental assumption of many advance statistical techniques. The skewness and kurtosis analysis can provide some idea with regard to the normality. Positive skewness values suggest clustering of data points on the low values (left hand side of the bell curve) and negative skewness values suggest clustering of datapoints on the high values (right hand side of the bell curve).
Positive kurtosis values suggest that the datapoints have peaked (gathered in centre) with long thin tails. Kurtosis values below 0 suggest that the distribution of datapoints is relatively flat (i.e. too many cases in the extreme). In a way, without normality and outliers assessment researcher may get false results which might lead to wrong conclusion and decision making.
KTL_003_C7_14: List the steps for generic hypothesis testing procedure.
Testing for statistical significance follows a relatively well-defined pattern, although authors differ in the number and sequence of steps. The generic process is described below.
1. Formulate the hypothesis
2. Select an appropriate test
3. Select desired level of significance
4. Compute the calculated difference value
5. Obtain the critical value
6. Compare the calculated and critical values
7. Marketing research interpretation